top of page
Key scientific publications
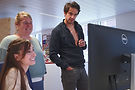
Built on Evidence. Proven in Practice.
We don’t guess. We validate, verify, and build with clinical rigor - delivering AI you can trust.
Backed by peer-reviewed science and real-world results.

Case studies & webinars
bottom of page